Brain-machine interfaces
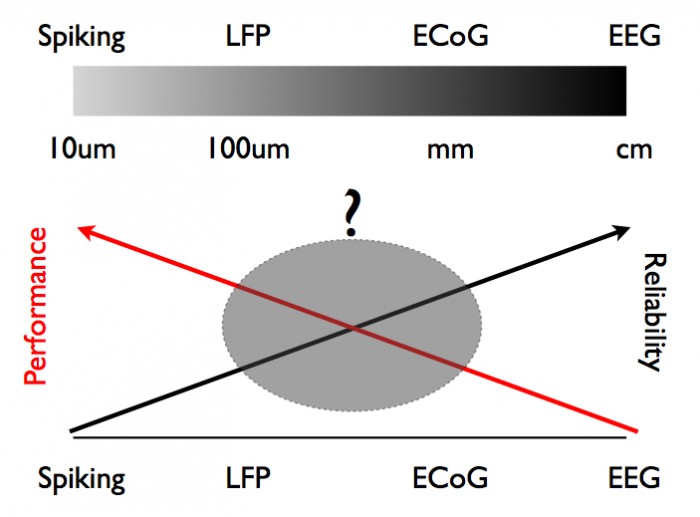
A brain-machine interface (BMI) records neural activity from the brain and processes it to generate a control signal. Neural activity recorded in the motor system, for example, can be used to move a prosthetic device such as a computer interface, wheelchair or a robotic arm. The ideal brain-machine interface should be reliable and support high-performance control. However, neural signals can be measured in many different ways (see Sidebar). Neural signals differ in the performance and reliability (see Figure 1). Spiking activity can support high-performance control but is difficult to obtain reliably. In contrast, neural activity measured at larger scales (LFP/ECoG) offers less detailed control than spiking, but may be more reliable. We are interested in understanding how to optimize BMI performance and reliability by incorporating neural signals at different spatial scales. We have been comparing the information contained in different neural signals. We have also recently engineered a new device which allows us to record spiking, LFP and ECoG signals at the same time from the same group of neurons.
Another direction in BMI is inspired by the success of Deep-Brain Stimulation. Stimulation-based BMIs process neural activity to control patterned brain stimulation. These systems close-the-loop between stimulation and recording to correct abnormal brain activity patterns. This approach has potential to treat a wide-range of neurological disorders (see Brain Stimulation).
Lab Researchers
Amy Orsborn, Kyle Brubaker, David Markowitz, David Putrino, Joshua Seideman, Adam Weiss, Yan Wong
Collaborators
John Rogers, Jonathan Viventi, Iahn Cajigas, Maryam Shanechi
Papers
Modeling multiscale causal interactions between spiking and field potential signals during behavior
Wang et al. (2022) Journal of Neural Engineering
Flexible, high-resolution thin-film electrodes for human and animal neural research
Chiang et al. (2021) Journal of Neural Engineering
Chiang et al. (2020) Science Translational Medicine
Parsing learning in networks using brain–machine interfaces
Osborn, Pesaran (2017) ClinicalKey
Utilizing movement synergies to improve decoding performance for a brain machine interface
Wong et al. (2013) Conf Proc IEEE Eng Med Biol Soc
Optimizing the decoding of movement goals from local field potentials in Macaque cortex
Markowitz et al. (2011) J Neurosci
Selecting the signals for a brain-machine interface
Andersen et al. (2004) Curr Opin Neurobiol
Project Funded By
DARPA Biological Technologies Office